AI Benchmarking: Shine a Light – Let’s Stop Planning in the Dark
I’m going to talk about something called AI Benchmarking. And to be clear, I’m not talking about traditional, tired old project benchmarking here – I am talking planning 2.0. I am talking about having my very own personal planning assistant. I am talking about having a virtual interactive planning session as I build my plan. I am talking about calibrating my plan against expert knowledge in real-time, to ensure realism and achievability.
-Dr. Dan Patterson
Traditional Project Benchmarking Is Not What I Am Talking About
Benchmarking means a lot of things to a lot of people – not to me! Traditional benchmarking involves taking your schedule and running comparisons against it. You might be comparing costs or comparing changes since your last schedule update. Traditional benchmarking is very useful for sure, but arguably not true benchmarking, given you are doing nothing more than a simple comparison, rather than truly undergoing informed validation and guidance.
Organizations have had the ability to compare plans for years and have traditionally called that benchmarking. This static process has been largely ineffective because each project has unique characteristics, and in the past, this has made it difficult to compare one plan to another or to a standard. For example, a hospital build project for a structure with 250 beds requires more scope than a hospital build with 100 beds.
Additionally, one of the major shortfalls of CPM tools is that it’s still a bit of a minefield when it comes to building a sound schedule – they simply don’t offer useful guidance. For many years, my mantra was “no constraints and no open ends in any schedule, period.” Well, let’s just say, I’ve evolved a bit in recent years. While I still believe in establishing a structurally sound plan, there are instances where the use of frowned-upon planning entities such as constraints (e.g., contractual windows) and even some open-ended logic (e.g., reporting milestones) is perfectly okay. What I am driving at here is that there isn’t sufficient guidance given when building a CPM plan. Sure, we have simple mathematical checks such as the 14-point assessment and so forth, but they do nothing for driving realism. This is where Artificial Intelligence can play a huge role in project planning today (more on this in a bit).
So, what I am driving towards is holistic planning guidance through the use of intelligent suggestions from the planning tool itself. Intelligent suggestions are backed up and supported by contextually relevant historical standards or benchmarks. “Don’t tell me my duration needs to be 45 days unless you can give me a reason why.” Suggestions need to be given with knowledge of context – context is key. When benchmarking a duration or a cost, or making a suggestion regarding sequence of work, having context of the quantities involved, or the location of the project, or the current market conditions is the difference between the suggestion being useful and completely worthless.
Interactive Planning Sessions Haven’t Helped Much Either
To help overcome this “every man for himself” nature of CPM planning, many organizations have adopted Interactive Planning Reviews. These sessions involve getting the project team together in a room to build a plan, identify project risk factors, and generally try and drive towards some degree of consensus. There are even so-called “Interactive Planning Tools” to try and facilitate these sessions, although many still prefer to use whiteboards and sticky notes!
There are multiple challenges with these interactive planning sessions. Firstly, they tend to be long and tedious, taking the entire project team way from their day job. Secondly, they don’t lend themselves to being consensus-driven or dare I say it – an open democracy. All too often, the loudest, most senior team member’s opinion sticks. Thirdly, those that carry the inherent knowledge as to how to execute a project typically aren’t CPM schedulers. They are construction managers, engineers, or discipline leads. There is a disconnect between those carrying the project knowledge and those responsible for putting a plan together.
So How Does Knowledge-Driven Planning Overcome These Shortfalls?
To make this simple, I will break down the concept and reality of Knowledge-Driven Planning into three simple steps:
- Calibrate: ensure your durations, costs, risks, and logic in your plan are relevant to what you are actually going to build during execution
- Validate: get buy-in from your team that what you have planned is indeed achievable
- Score: quantify the realism of your plan
Calibrate Through Benchmarking
One of the most useful capabilities of Knowledge-Driven Planning is the real-time guidance it provides; real-time when developing a new plan from scratch as well as real-time when reviewing an existing plan during interactive planning sessions.
As you walk through the plan, suggestions as to recommended durations, which activities should be included, logic between activities, and even costs are given. This is achieved through an always-on Artificial Intelligence engine that sits behind the scenes. This engine makes suggestions based on a knowledge-library containing historical benchmarks, but the real magic is in the fact that it understands context.
In the example below, we are establishing our Detailed Engineering plan, specifically for the “Topsides” scope of the project. “Topsides Detailed Engineering” includes three activities (“Upper Deck Design,” “Lower Deck Design,” and “Turret Design”). We have established durations and sequence of work, and as we are doing so, the AI engine has returned suggestions based on historical benchmarks. On the right-hand side, we can see that “Topsides Detailed Engineering” typically takes not 256 days, but instead 212 days. But there is more, that benchmark of 212 days is based on the delivery of 72 drawings. Our project only involves 50 drawings, and so the AI engine has gone a step further and factored this benchmark, adjusting for the difference in quantity to normalize the suggestion to an equivalency of 147 days.
From this point forward, updating our plan to fit the adjusted suggestion is as simple as clicking on the waypoint marker. Boom! We now have an accurate plan for “Topsides Detailed Engineering” that has been calibrated using historical benchmarks, but most importantly, there is intelligence behind this suggested benchmark – it isn’t just a basic comparison from a database. The engine also has a sense of realism in that if I am within a reasonable tolerance, then I don’t get dinged as being unrealistic. The AI engine really does think like a reasonable human!
The suggestion has taken into account context of the type of work as well as the scope involved. I am even prompted if there are missing activities within my “Topsides Detailed Engineering” scope that I perhaps forget beyond the three that I defined.
Validate Through Team Member Buy-In
Having a plan that has been calibrated by a very clever AI assistant is one thing, but does your team really believe it is achievable? This is where team member buy-in comes into play – we call this Human Intelligence.
Capturing expert opinion from multiple team members and then establishing a consensus is an extremely powerful way of determining whether your team believes in the plan or not.
Take this consensus view and compare it back to your AI benchmark-driven plan and you get the best of both worlds:
- Assurance that what you have built is realistic and aligns with historical benchmarks
- Validation that your project team is bought into this plan and stand behind it
What is key when capturing team member buy-in is to keep it meaningful, avoiding overwhelming team members with unnecessary CPM jargon. This process should be as simple as, “Do you buy into the plan, yes or no?” “If no, tell me what you think.”
Don’t confuse team members with complex Gantt charts showing early, late, target, baseline, and contract dates, for example. Team members generally understand start and end of an activity and don’t really care that behind the scenes there is a calendar tied to the working duration that is impacting early and late dates. (That is all CPM-stuff that a planner needs to understand, not a team member.) Do you need to understand the underlying file format of a MS Word document to be effective when using MS Word? Of course not. The same applies to CPM scheduling when interviewing team members.
Score Your Project Plan Realism
Having a means of measuring the realism of your plan helps drives you to an end goal. It gives you insight as to whether your plan is good enough or whether you need to keep working it to get it to a more realistic state.
To achieve this, we have developed the Basis Realism Index. This index is a zero to ten score that reflects how much of your defined scope in the plan is realistic. Of course, the higher the score, the more realistic the plan, and the better chance of achieving it during execution.
Additional supporting measures such as “Detail” tell us whether we are missing detail in certain areas of the plan, and “Continuity” is a measure of how many gaps there are in the plan that could be used to improve the flow of work.
Integration with Scheduling Tools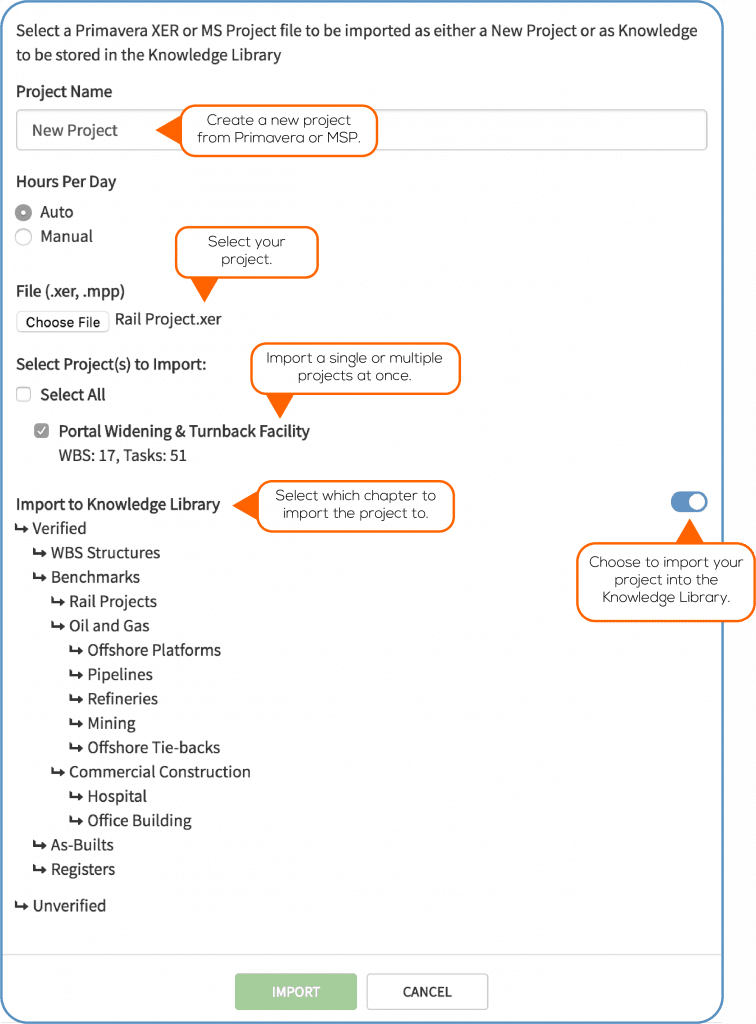
Creating a plan in InEight Basis is as simple as starting from scratch, leveraging the Knowledge Library and InEight Basis shortcuts allowing for plans to be created quickly. Or, alternatively, bring in an existing plan from Primavera or Microsoft Project. Existing plans can also be imported directly into your organization’s Knowledge Library allowing you to house history, standards and benchmarks in a single location.
When you bring in a plan to InEight Basis from an XER you can choose to import all projects contained within that file or select only a single project. In addition, InEight Basis supports not only the legacy global hours per day definition from Primavera, but also the more recent addition of activity-level hours per day assignments.
Once the plan is a part of InEight Basis, utilize Smart Planning capabilities to further flesh out your work and benchmark your plan against the information resident in your Knowledge Library.
When you are ready to push your plan to Primavera or Microsoft Project you can choose to export the project in its entirety or select which portion of the plan you would like to export. When exporting to Primavera, InEight Basis is intelligent enough to only export the changes allowing project creation in Primavera but also incremental schedule updates without the need for complicated merging.
Conclusion
Please don’t think I am for replacing CPM. Far from it. What I am pushing for though is for CPM to evolve. Evolve such that scheduling tools start to be pro-active in guiding us through the planning process, rather than just being a static drawing board onto which you can freely draw anything.
The recent advent of AI in mainstream computing has allowed us to take a huge step forward in taking this vision of CPM tools being proactive and offering informed suggestions to reality.
Today, with the release of InEight Basis, we are already there. InEight Basis combines AI benchmarking with team member markup (Human Intelligence or HI) to drive towards more realistic plans. Quantifying realism through the Realism Index also gives us a means of tracking improvement (e.g., is our realism increasing) and how we compare to other projects.
For more information about InEight Basis’ planning and scheduling solutions, visit ineight.com/contact.